Author: Qin Jin; Source: Carbon Chain Value
What Silicon Valley tycoons and Wall Street elites never expected was that a mysterious force from the East, DeepSeek, could actually cause the US stock market and its huge wealth to fall and shrink overnight. On January 28, according to relevant data, US chip stocks evaporated $1 trillion overnight. Nvidia's market value evaporated $600 billion in a single day, setting a record for the US stock market. Even US President Trump couldn't help but make a comment. He said that the sudden rise of China's artificial intelligence application DeepSeek "should sound the alarm for American technology companies" and said it was a good thing that Chinese companies had developed cheaper and more efficient artificial intelligence models.
Trump said he still expects American technology companies to dominate the field of artificial intelligence, but he acknowledged the challenges posed by the low-cost artificial intelligence assistant DeepSeek. DeepSeek soared to the top of the Apple App Store over the past weekend.
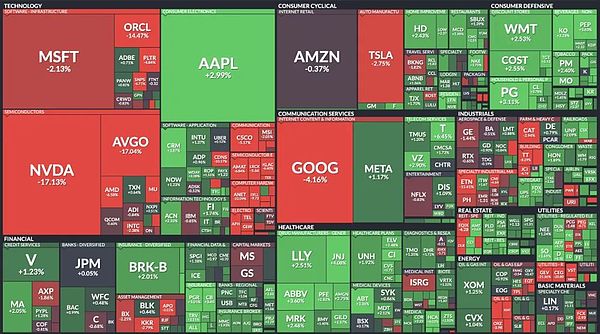
The next question is, is this just the beginning?
On January 28, the Financial Times reported that a little-known Chinese hedge fund had dropped a bombshell on the field of artificial intelligence. The large artificial intelligence model they developed is actually on par with the market leader Sam Altman's OpenAI, but the cost is only a fraction of the latter. OpenAI regards the working principle of its model as proprietary technology, while DeepSeek's R1 has open-sourced the core technology, thus attracting developers to use and develop on it.
Before the opening of the U.S. stock market on Monday, the market value of the five largest technology stocks in the field of artificial intelligence - Nvidia, Alphabet, Amazon, Microsoft and Meta Platforms - evaporated by a total of nearly $750 billion. If DeepSeek really wins without using its most advanced chips, it could be particularly tough for Nvidia.
After the U.S. stock market opened: Chip stock Nvidia fell as much as 17.5%. Broadcom fell as much as 16.5%. TSMC fell 14.3%. Micron fell 10.5%. Arm fell 10.0%. AMD fell 5.6%. The above chip stocks lost nearly $1 trillion in market value in one day. Among them, Nvidia has lost all its gains since October 2, falling 21% in two trading days. Nvidia's market value has fallen by $630 billion. It was the world's largest listed company last week, and it lost one-fifth of its value in two days. Huang Renxun's personal worth has also shrunk by $100 billion.
Investors in technology and energy companies, including chipmaker ASML, who had hoped that the development of data centers would drive corporate performance growth, are now worried that their investments will go to waste. According to Visible Alpha estimates, super-large companies will invest nearly $300 billion in capital expenditures this year. Analysts expect Meta and Microsoft to report a total investment of $94 billion in 2024 when they announce earnings on Wednesday.
Some foreign media analysis said that the artificial intelligence race has begun. The reasons for the collapse of Nvidia's stock price obviously include that DeepSeek has become a leader in artificial intelligence innovation, which Nvidia calls "excellent artificial intelligence progress."
Secondly, in theory, artificial intelligence progress is good for Nvidia because it means more chip demand. The problem is that DeepSeek is in China, and the United States is blocking China's chip technology. This means that Nvidia can only sell DeepSeek a large number of its less powerful H800 chips, rather than its H100 chips, which cost more than $30,000 each. Even if DeepSeek only holds on to the lower-performing chips, China has effectively been able to produce an AI model that costs 96% less and is more efficient, and the price is more than 90% lower.
At the same time, their AI model is free, while AI models like ChatGPT are relatively expensive ($200+ per month) and less efficient. It seems that China has begun to innovate at a rate that was previously thought impossible. The US tech community believes that its dominance cannot be replaced. US large-cap tech stocks are now facing the reality that they can get better AI models for free, and they are only getting better. Why pay a premium for an inferior product?
The next response may be that the US restricts DeepSeek or exports of chips to China (which we have seen for months), but this obviously does not work. If Nvidia can find a way to take advantage of the new demand brought about by DeepSeek's development, then this decline will be seen as a gift. Ultimately, competition in AI hardware and software will intensify, no company will be "safe", and the pace of innovation will only accelerate.
So what is the reason for DeepSeek's victory? And how will AI unfold in the future?
Gavin Baker, managing partner of the US investment company Atreides, analyzed DeepSeek and the future evolution trend of AI from multiple dimensions. Musk praised this as the best analysis he had ever seen.
Gavin Baker said that most importantly, DeepSeek R1 is much cheaper than Open ai o1 and has higher inference efficiency, which is not derived from the $6 million training data. R1 costs 93% less to use per API than o1, can run on local high-end workstations, and does not seem to reach any crazy rate limits. Simple math shows that in FP8, each 1b of active parameters requires 1gb of RAM, so R1 requires 37gb of RAM. Batching can greatly reduce costs, while more compute can increase tokens per second, so there is still an advantage to doing inference in the cloud. Also note that there are indeed geopolitical dynamics at play here, which I don't think is coincidental since this is after Stargate. $500 billion - we barely even know you.
DeepSeek is/was #1 in downloads in the relevant category on the App Store. Clearly ahead of ChatGPT; something neither Gemini nor Claude can do. 2) From a quality perspective, it is on par with o1, though behind o3. 3) There have been real breakthroughs in algorithms that have made training and inference much more efficient. Training for FP8, MLA, and multi-label predictions is non-trivial. 4) It's easy to verify that the r1 training run only cost $6 million. While this is true, it's also deeply misleading. 5) Even their hardware architecture is novel, and I'll note that they use PCI-Express for scaling.
Nuances: 1) According to the technical paper, the $6 million does not include "costs associated with prior research and ablation experiments on architectures, algorithms, and data." This means that it is possible to train an r1-quality model with a running cost of $6 million if the lab has spent hundreds of millions of dollars on prior research and has access to larger clusters. Deepseek apparently has more than 2,048 H800s; one of their earlier papers mentioned a cluster of 10,000 A100s. There is no way a similarly smart team could have built a cluster of 2,000 GPUs for only $6 million and trained r1 from scratch. About 20% of Nvidia's revenue comes from Singapore. Despite Nvidia's best efforts, 20% of the company's GPUs are probably not in Singapore. 2) They did a lot of refining work - that is, it is unlikely that they could have trained this model without unfettered access to GPT-4o and o1. As @altcap pointed out to me yesterday, it’s a bit funny to restrict access to leading GPUs while doing nothing to address China’s ability to refine leading US models — which clearly defeats the purpose of export restrictions. Why buy cows when you can get milk for free?
Conclusions: 1) Lowering training costs will improve AI ROI. 2) This is not positive for training capex or the “power” theme in the short term. 3) The biggest risk to the current winners of “AI infrastructure” in tech, industrial, utilities, and energy is that a stripped-down version of the r1 can run locally at the edge on a high-end workstation (someone mentioned the Mac Studio Pro). This means similar models will be running on super phones in about two years. If inference moves to the edge because it’s “good enough”, we live in a very different world with very different winners — namely the biggest PC and smartphone upgrade cycle we’ve ever seen. Computing has been oscillating between centralization and decentralization for a long time. 4) ASI is really, really close, and no one really knows what the economic rewards of super intelligence will be. If a $100 billion inference model trained on 100,000+ Blackwells (o5, Gemini 3, Grok 4) cures cancer and invents warp drives, then the payback for ASI will be very high, and training cost expenditures and power consumption will steadily increase; Dyson spheres will re-emerge as the best explanation for the Fermi Paradox. I hope the payback for ASI is high - that would be awesome. 5) This is really good for companies that use AI: software, the internet, etc. 6) From an economic perspective, this greatly increases the value of distribution and unique data - YouTube, Facebook, Instagram, and X. 7) US labs may stop releasing their leading models to prevent the refining process that is essential in R1, although it may be fully exposed in this regard. ie R1 may be enough to train R2, etc.
Grok-3 is coming out soon and may have a significant impact on the above conclusions. This can be said to be the first major test of the law of scale in pre-training since GPT-4. Just as it took weeks to turn v3 into r1 via reinforcement learning, the reinforcement learning required to improve Grok-3's inference capabilities will likely take weeks. The better the base model, the better the inference model should be, because the three scaling laws are multiplicative - pre-training, reinforcement learning during post-training, and test-time computation during inference (a function of reinforcement learning). Grok-3 has demonstrated that it can accomplish tasks beyond o1 - see the Tesseract demo - and how far it can go will be important. To paraphrase an unnamed orc in The Two Towers, maybe it won't be long before we can eat meat again. Time will tell, "Facts speak louder than words, and I will change my mind." However, US stock investment analyst Xu Laomao believes that Monday's sell-off due to DeepSeek was an overreaction. The long-term growth narrative of AI remains intact and arguably stronger now than ever. There is a Jevons paradox in economics, which states that increased efficiency in resource utilization tends to increase (rather than decrease) the overall consumption of that resource, because higher efficiency reduces the cost of using the resource, leading to an increase in demand for the resource.
This happened with the use of coal in steam engines. Increased efficiency of steam engines reduced coal consumption per unit of output. However, it also made coal-fired technology more economically attractive, leading to wider adoption, which ultimately increased overall coal consumption. This happened on the Internet, as computers became smaller and cheaper, and Internet access was basically free, everyone had a computer, and everyone had 24/7 access to the Internet.
The same is true for AI now. As the cost of training and inference of AI models has dropped significantly, more and more companies and individuals will build their own AI models, and AI will become popular and penetrate into all aspects of society around the world.