Author: Poopman, Ouro Research; Translation: 0xjs@黄金财经
FHE opens the possibility of computing on encrypted data without decryption.
When combined with blockchain, MPC, ZKP (scalability), FHE provides the necessary confidentiality and supports a variety of on-chain use cases.
This article briefly outlines the current status of FHE.
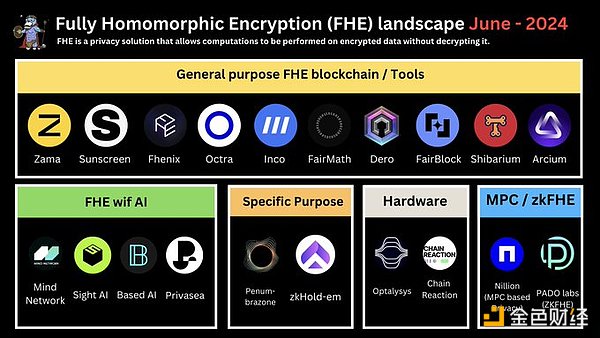
I will introduce: 1. FHE background; 2. How does FHE work? 3. 5 areas in the FHE ecosystem; 4. Current FHE challenges and solutions.
1. Background
FHE was first proposed in 1978, but due to its high computational complexity, it was not practical for a long time and remained only at the theoretical level.
It was not until 2009 that Craig developed a feasible model for FHE, and since then, research interest in FHE has soared.
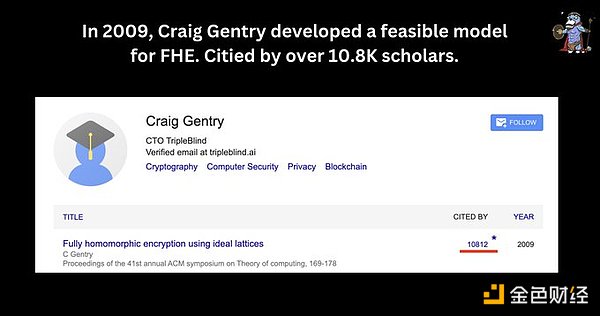
In 2020, Zama launched TFHE and fhEVM, making FHE the focus of attention in the encryption field.
Since then, we have seen the emergence of general EVMs compatible with FHE L1/L2 such as Fhenix, Inco Network, and FHE compiler Sunscreen Tech.
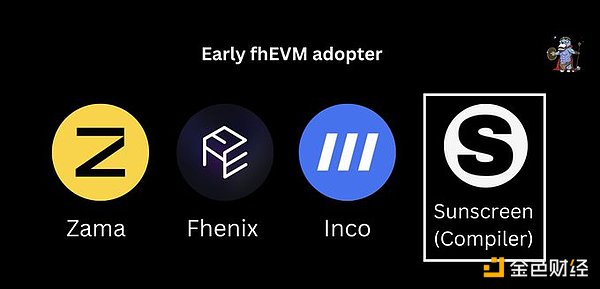
How does FHE work?
You can imagine a blind box with a puzzle inside. However, the blind box cannot know anything about the puzzle you gave it, but it can still calculate the result mathematically.
Some use cases of FHE include: private on-chain computing, on-chain data encryption, private smart contracts on public networks, confidential ERC20, private voting, NFT blind auctions, more secure MPC, front-running protection, trustless bridges, etc.
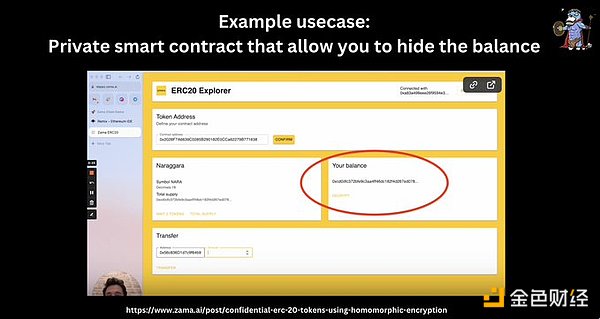
III. FHE Ecosystem
Overall, the landscape of on-chain FHE can be summarized into 5 areas: 1. General FHE; 2. FHE/HE for specific use cases (applications); 3. FHE acceleration hardware; 4. FHE with AI; 5. Alternative solutions
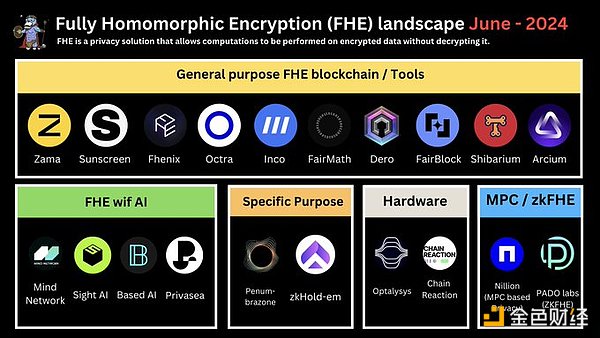
1. General FHE blockchain and tools
They are the backbone to achieve blockchain confidentiality. This includes SDK, coprocessor, compiler, new execution environment, blockchain, FHE module...
The most challenging one is: introducing FHE into EVM, namely fhEVM.
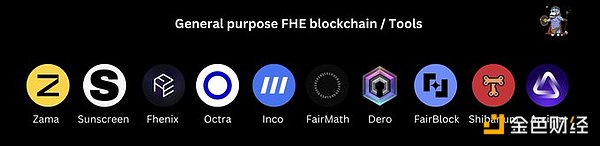
Including:
fhEVM: Zama, Fhenix, Inco network, Fair Math
FHE tools/infrastructure: Octra, Sunscreen Tech, Fairblock, Dero, Arcium (formerly Elusiv), Shibarium
Below is a one-sentence summary of each project.
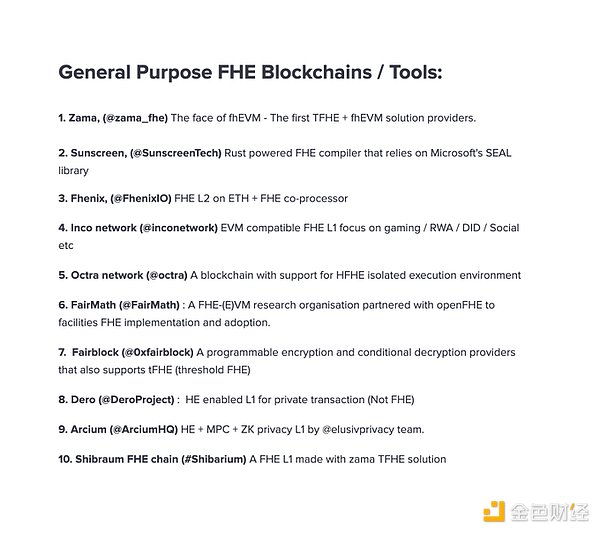
2. FHE/HE for special-purpose applications
Penum: Cross-chain cosmos dex (appchain) using tFHE for shielded exchange/pool.
zkHoldem: Poker game on Manta Network, using HE and ZKP to prove the fairness of the game.
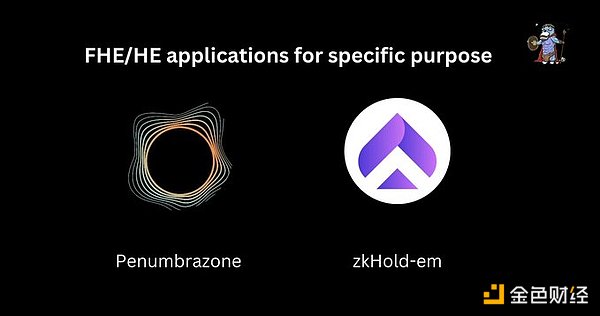
3. Acceleration Hardware
Whenever FHE is used for intensive computations such as FHE-ML, guidance to reduce noise growth is critical. Solutions such as hardware acceleration play an important role in facilitating guidance, with ASICs performing best.
Members in the hardware field include: Optalysys, Chain Reaction, Ingonyama, Cysic
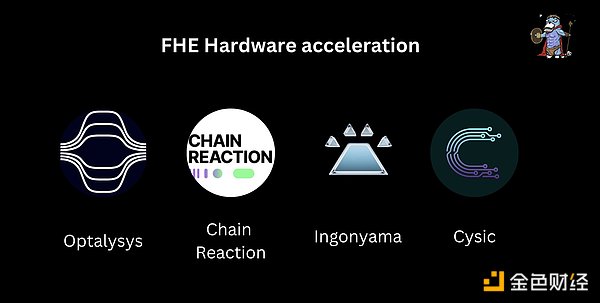
Each company specializes in producing products that can accelerate FHE Hardware for boot/computation, such as chips, ASICs, and semiconductors.
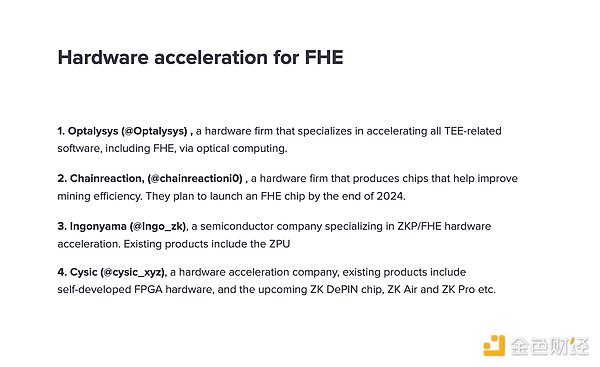
4. AI X FHE
Recently, there has been a growing interest in integrating FHE into AI/ML. Among them, FHE can prevent machines from learning any sensitive information when processing sensitive information, and provide confidentiality for data, models, and outputs throughout the process.
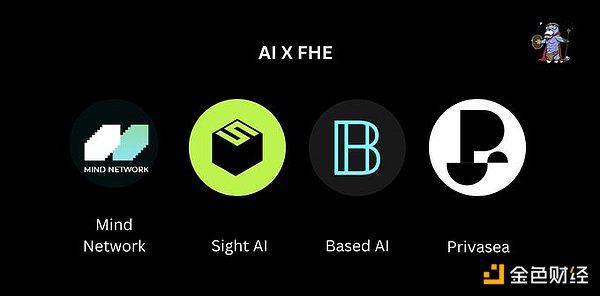
Members of AI x FHE include: Mind Network, Sight AI, BasedAI, Privasea
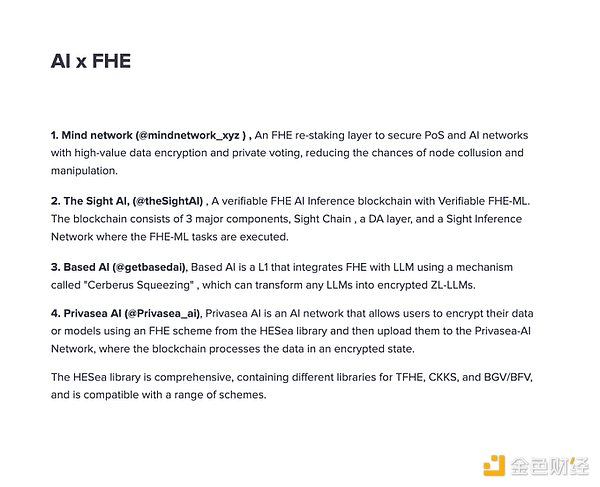
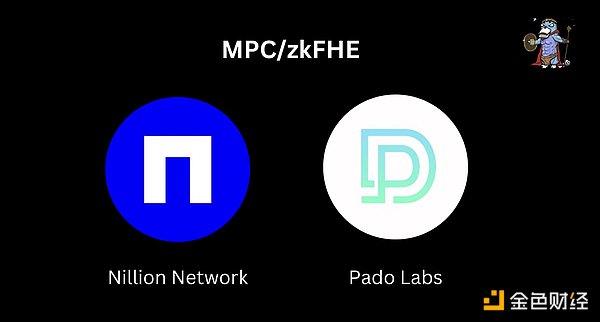
They are: Nillion network, Pado Labs
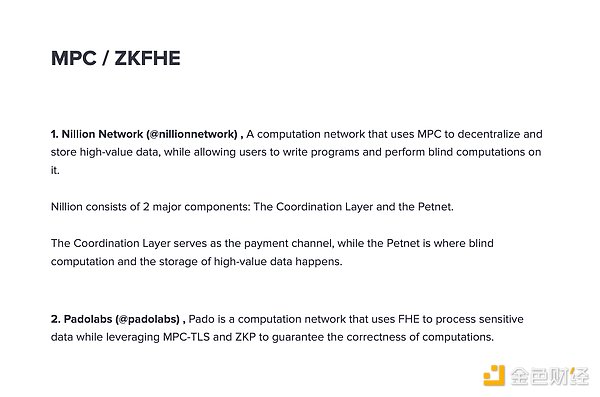
Fourth, FHE challenges and solutions
With ZK and MPC Unlike traditional cryptographic algorithms, FHE is still in its early stages.
Major challenges include:
Slow performance: Currently, private smart contracts using fh-EVM have only 5 TPS. In addition, TFHE is now about 1000 times slower than pure data.
Not developer-friendly yet: There is still a lack of standardized algorithms and overall supported FHE tools.
High computational overhead (cost): It can lead to node centralization due to noise management and bootstrapping of complex computations.
Risks of insecure on-chain FHE: For any secure threshold decryption system, the decryption keys are distributed among nodes. However, due to the high overhead of FHE, this may lead to a reduced number of validators and therefore a higher probability of collusion.
Solutions:
Programmable bootstrapping: It allows computation to be applied during bootstrapping, thereby improving efficiency while being application-specific.
Hardware acceleration: ASICs, GPUs, and FPGAs are being developed together with the OpenFHE library to accelerate FHE performance.
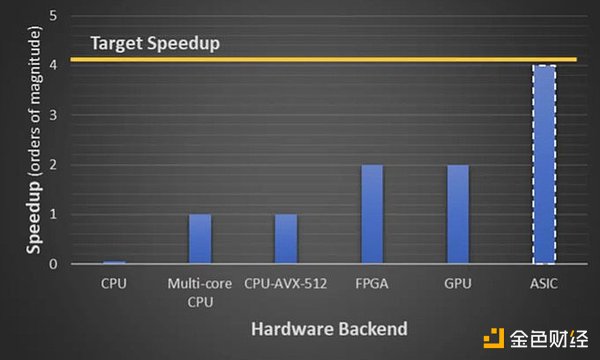
Better threshold decryption system: In short, in order to make on-chain FHE more secure, we need a system (can be MPC) to ensure: low latency, lower decentralized entry barriers for nodes, and fault tolerance.