1. The Prospect of Centralized AI Agents
AI agents have the potential to revolutionize the way we interact with the web and perform tasks online. While there has been a lot of discussion around AI agents leveraging cryptocurrency payment rails, it is important to recognize that established Web 2.0 companies are also well positioned to offer comprehensive agent (AI agent) product suites.
Agents from Web2 companies are mostly in the form of assistants or vertical tools with only weak execution capabilities. This is due to both the immaturity of the underlying model and regulatory uncertainty. Today's agents are still in the first stage, they can do well in specific areas, but have little generalization capabilities. For example, Alibaba International has an agent that specializes in helping merchants reply to emails regarding credit card disputes. It is a very simple agent that calls up data such as shipping records, generates and sends them according to templates, and has a high success rate that allows credit card companies to not deduct money.
Tech giants like Apple and Google, and AI expert companies like OpenAI or Anthropic, seem particularly well suited to explore the synergies of developing agent systems. Apple's advantage lies in its ecosystem of consumer devices, which can serve as a host for AI models and a portal for user interaction. The company's Apple Pay system can enable agents to facilitate secure online payments. Google, with its vast web data index and ability to provide real-time embedding, can provide agents with unprecedented access to information. At the same time, AI powerhouses like OpenAI and Anthropic can focus on developing specialized models that can handle complex tasks and manage financial transactions. In addition to Web2 giants, there are also a large number of startups in the United States that do such agents, such as helping dentists manage appointments or assisting in generating post-treatment reports, which are very detailed scenarios.
However, these Web 2.0 giants face the classic Innovator's dilemma. Despite their technological strength and market dominance, they must navigate the dangerous waters of disruptive innovation. Developing truly autonomous agents represents a major deviation from their established business models. In addition, the unpredictable nature of AI, combined with the high stakes of financial transactions and user trust, presents significant challenges.

2. The Innovator’s Dilemma: Challenges for Centralized Providers
The Innovator’s Dilemma describes a paradox whereby successful companies often have difficulty adopting new technologies or business models, even when those innovations are critical to long-term growth. At the heart of the problem is the reluctance of existing companies to introduce new products or technologies whose initial user experience may not be as sophisticated as their existing products. These companies fear that adopting such innovations may alienate their current customer base, who have become accustomed to a certain level of sophistication and reliability. This hesitation stems from the risk of undermining the expectations that users have long nurtured.
2.1 Agent Unpredictability and User Trust
Large tech companies like Google, Apple, and Microsoft have built their empires on proven technologies and business models. Introducing fully autonomous agents represents a significant departure from these established norms. These agents, especially in their early stages, will inevitably be imperfect and unpredictable. The non-deterministic nature of AI models means that there is always a risk of unexpected behavior even after extensive testing.
For these companies, the stakes are very high. A misstep could not only damage their reputation, but could also expose them to significant legal and financial risk. This creates a strong incentive for them to proceed with caution, potentially missing out on first-mover advantages in the agent space.
For centralized providers considering deploying agents, the risk of customer protests is very high. Unlike startups that can pivot quickly and without much to lose, established tech giants have millions of users who expect consistent, reliable service. Any major misstep by an agent can result in a PR nightmare.
Consider a scenario where an agent makes a series of poor financial decisions on behalf of a user. The resulting outcry could erode years of carefully built trust. Users may question not only the agent, but all of the company’s AI-based services.
2.2 Ambiguous Evaluation Criteria and Regulatory Challenges
Further complicating the issue is how to evaluate what is a “correct” agent response. In many cases, it’s unclear whether the agent’s response was truly incorrect or just an accident. This gray area can lead to disputes and further damage customer relationships.
Perhaps the most daunting hurdle facing centralized agent providers is the evolving and complex regulatory landscape. As these agents become more autonomous and handle increasingly sensitive tasks, they enter a regulatory gray area that can pose significant challenges.
Financial regulations are particularly thorny. If an agent makes financial decisions or executes trades on behalf of a user, it may be regulated by financial regulators. Moreover, compliance requirements may be extensive and vary significantly across jurisdictions.
There is also the question of liability. If an agent makes a decision that results in financial loss or other harm to a user, who should be held responsible? The user? The company? The AI itself? These are questions that regulators and lawmakers are just beginning to grapple with.
2.3 Model bias can be a source of controversy
In addition, as agents become more sophisticated, they may run afoul of antitrust regulations. If a company’s agents consistently favor that company’s own products or services, this could be seen as anticompetitive behavior. This is particularly important for tech giants, which are already under scrutiny for their market dominance.
The unpredictability of AI models adds another layer of complexity to these regulatory challenges. When Web2 cannot fully predict or control the behavior of AI, it is difficult to ensure compliance with regulations. This unpredictability may lead to slower innovation in Web2 agents as companies need to deal with these complex issues, which may give advantages to more flexible Web3 solutions.

3. Opportunities for Web3
As the capabilities of the underlying LLM model improve, agents have the opportunity to enter the next form, agents with relatively high autonomy. At present, it seems unlikely that large companies will dare to touch this aspect. Maybe helping users order a pizza is the limit. Startups may be bold, but they will face many technical obstacles. For example, the agent itself does not have an identity. Any operation needs to borrow the identity and account of the agent user. Even if the identity is borrowed, it is not so easy for the traditional system to support the agent to operate freely. Web3 technology provides a unique opportunity for the development of artificial intelligence agents, which may solve some challenges faced by centralized providers. Under the Web3 system, agents can realize multiple DIDs by mastering wallets. Whether it is payment through encryption or the use of various permissionless protocols, it is very friendly to agents. When agents begin to carry out complex economic behaviors, there is a high probability that agents and agents will have high-intensity interactions. At this time, if the mutual suspicion between agents cannot be resolved, the agent economic system is not a complete economic system. This is also an aspect that can be solved by using encryption technology.
In addition, Crypto-economic incentives can promote agent discovery and provide a penalty that can be reduced or confiscated if the agent behaves improperly. This creates a self-regulating system where good behavior is rewarded and bad behavior is punished, thus potentially reducing the need for centralized oversight and providing a level of peace of mind to those early adopters of delegating financial transactions to fully autonomous agents.
Crypto-economic staking serves a dual purpose, being slashed in case of misbehavior, and also acting as a key market signal in the agent discovery process. The intuition is simple, both for other agents and for people looking for a specific service, that the more staking, the higher the market trust in a particular agent’s performance, and the more peace of mind users will have. This could create a more dynamic and responsive agent ecosystem, where the most efficient and trustworthy agents naturally rise to the top.
Web3 also enables the creation of open agent markets. These markets allow for a greater degree of experimentation and innovation than would be possible with trusting a centralized provider. Startups and independent developers can contribute to the ecosystem, potentially leading to faster advancement and professionalization of agents.
In addition, distributed networks like GRASS and OpenLayer can provide agents with the opportunity to access both open Internet data and closed information that requires authentication. This broad access to diverse data sources may enable Web3 agents to make more informed decisions and provide more comprehensive services.
Web 2.0 vs. Web 3.0
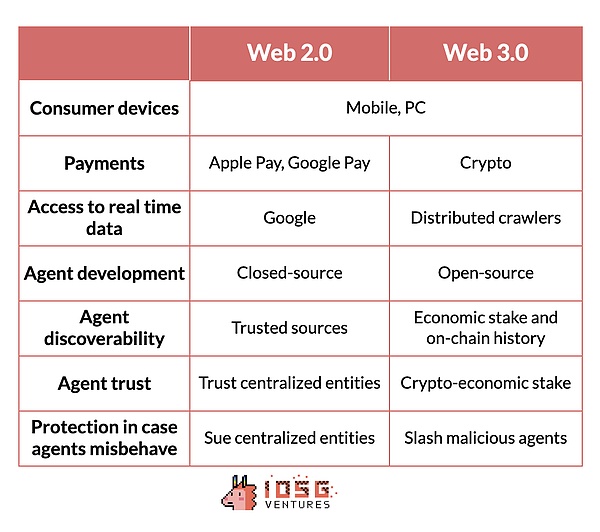

4. Limitations and Challenges of Web3 AI Agents
4.1 Limited Adoption of Crypto Payments
This post would not be complete if we did not reflect on some of the adoption challenges that Web 3.0 agents will face. The elephant in the room is that the adoption of cryptocurrencies as a payment solution for the off-chain economy is still limited. Currently, only a handful of online platforms accept crypto payments, which limits the practical use cases of crypto-based agents in the real economy. Without deep integration of crypto payment solutions with the broader economy, the impact of Web 3.0 agents will continue to be limited.
4.2 Transaction Size
Another challenge is the size of typical online consumer transactions. Many of these transactions involve relatively small amounts of money, which may not be enough to justify a trustless system for most users. The average consumer may not see the value in using a decentralized agent for small, everyday purchases if a centralized alternative exists.

5. Conclusion
The unpredictability of non-deterministic models has led to the reluctance of technology companies to provide fully autonomous AI agents, creating opportunities for crypto startups. These crypto startups can use open markets and crypto-economic security to bridge the gap between agent potential and actual implementation.
By leveraging blockchain technology and smart contracts, crypto AI agents may provide a level of transparency and security that is difficult for centralized systems to match. This may be particularly attractive for use cases that require a high degree of trust or involve sensitive information.
In summary, while both Web2 and Web3 technologies provide avenues for the development of AI agents, each approach has its own unique advantages and challenges. The future of AI agents may depend on how effectively these techniques can be combined and refined to create reliable, trustworthy, and helpful digital assistants. As the field develops, we may see a convergence of Web2 and Web3 approaches, leveraging the strengths of each to create more powerful and versatile AI agents.