Source: Investment Internship
After the rise of this wave of AI, we often compare it with the previous Internet wave, thinking that the rise of AI companies is like the rise of Internet companies. My feeling is that there is actually a big difference between the two. AI gives me the feeling of being more centralized, while the Internet is more decentralized.
Recently, Marc Andreessen and Ben Horowitz, the two founders of a16z, just talked about this topic. They think that this analogy is not completely applicable. From the technical essence, Marc Andreessen believes that the Internet is a network, while AI is more like a computer.
01. Differences in the nature of technology
The Internet is essentially a network that connects many existing computers and promotes the creation of new computers. It is dominated by network effects. As more people join, the value of the network increases, and it prompts people to build more new computers to connect to the Internet.
AI, especially large language models, is a new type of computer, a probability-based, neural network-based computer, which is very different from the previous von Neumann-type computers (deterministic computers).
The development of AI is more similar to the era of microprocessors or mainframes. It processes data, learns from it, and generates output. It is an information processing system, not a network.
The first computers were very large and expensive, and people thought that only a few computers were needed in the world. But over time, computers became smaller and cheaper, and eventually became ubiquitous.
The development of AI will also follow a similar pattern, and there will eventually be AI models of various shapes, sizes, and capabilities, which will be trained on different types of data and run at different scales, with different privacy and security policies.
02. Differences in industry development
In the Internet era, everyone focused on building networks and applications that exploit network effects. Companies strive to gain and maintain user bases to take advantage of these effects.
In the AI era, the core involves building various AI models and applications, with a focus on improving the capabilities of these models and integrating them into different fields.
03. Lock-in and availability
Ben Horowitz believes that unlike previous computers, AI is the easiest computer to use so far because it can speak natural languages like English, just like talking to people.
This raises questions about the lock-in effect of AI, i.e., whether users are completely free to choose the size, price, speed of selection of the AI model they need, or whether they will be locked into a particular large model.
In the Internet era, lock-in was very important and switching costs were high due to the complexity of using early computers and networks.
In the AI era, AI is easier to use because it can understand and generate human language, which reduces lock-in and provides greater flexibility in choosing AI solutions.
04. Similarities between the two waves
First, there was a speculative boom and bust: Both waves experienced a speculative investment cycle, with initial excitement triggering overinvestment, followed by a crash when expectations failed to materialize. This cycle is typical for new, transformative technologies.
Economic and cultural impact: Both technologies have had a profound impact on the economy and culture. The Internet revolutionized communications, commerce, and information sharing.AI is expected to transform industries by automating tasks, enhancing decision-making, and creating new capabilities.
05. Lessons Learned
Boom and Bust: Cycles of overinvestment and correction are expected as a natural part of the adoption curve of transformative technologies.
Open vs. Proprietary Systems: The Internet started as a proprietary network and then moved to openness, which fueled its growth. There is a risk that AI will move toward a more closed system, which could stifle innovation and competition.
Speculative Investment: Speculative investment is a double-edged sword. It can lead to rapid development and deployment of new technologies, but can also lead to significant financial losses when expectations are not met.
06. Future Outlook
AI Models: The future of AI is likely to involve a diverse ecosystem of various sizes and models, similar in functionality to the evolution of computers from mainframes to microprocessors.
Integration and Applications: The focus will be on integrating AI into various fields and creating applications that leverage AI’s unique capabilities.
Below is a simple text translation of the conversation video, you can watch the original conversation video here (https://dub.sh/Memo2):
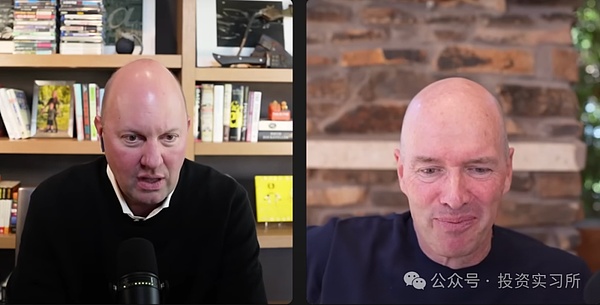
Marc Andreessen:What is the strongest common theme between the current state of AI and Web 1.0? Ben, let me give you a theory first and see what you think.
Because my role and your role at Netscape, we were involved in the development of the early Internet and I get asked this question a lot. So the Internet boom was a major event in the technology field and it's still in the memory of many people.
People like to reason from analogies, so they think the AI boom should be like the Internet boom, and starting an AI company should be like starting an Internet company. So what are the similarities between the two?
Actually, I think this analogy doesn't hold up in most cases. It may hold up in some ways, but it doesn't apply in most cases. The reason is that the Internet is a network, and AI is a computer.
Let's explain this idea. The PC boom, or the microprocessor boom, I think the best analogy is the microprocessor, even going back to the original computer, the mainframe era.
The reason is that the Internet is a network, it connects many existing computers, and of course, people have built many new computers to connect to the Internet. But fundamentally, the Internet is a network.
Most of the industry dynamics, competitive dynamics, and entrepreneurial dynamics about the Internet are related to building networks or applications that run on networks. Startups in the Internet era are very focused on network effects and the various positive feedback loops that arise from connecting a large number of people, such as the so-called Metcalfe's Law, which states that the value of a network increases as the number of users increases.
【 Memo Note: Metcalfe's Law is a network effect theory proposed by Robert Metcalfe. The law states that the value of a network is proportional to the square of the number of users on the network. Specifically, when the number of users in a network increases, each user can connect to more other users, and the overall value of the network will increase significantly. Metcalfe's law can be expressed in a simple formula: V ∝ n², where (V) represents the value of the network and n represents the number of users in the network. 】
AI also has network effects in some ways, but it is more like a microprocessor, more like a chip, more like a computer. It is a system, data goes in, data is processed, data goes out, and then things happen. It is a computer, an information processing system, and a new computer.
We like to say that computers so far are so-called von Neumann machines, that is, deterministic computers, they are very strict, they do exactly the same thing every time, and if they make a mistake, it is the programmer's fault. But they are very limited in interacting with people and understanding the world.
We think thatAI and large language models are a new type of computer, a probabilistic computer, a computer based on neural networks. They're less accurate, they won't give the same results every time, and they might even argue with you and not answer your question.
That makes them fundamentally very different from old computers, and makes it more complex to build large-scale systems, but their capabilities are new and different and valuable and important because they can understand language and images and do all the things you see when you use them.
So I think the analogies and lessons are more likely to come from the early days of the computer industry or the early days of the microprocessor than from the early days of the internet. Do you think that's right?
Ben Horowitz: I think that's right. Although that doesn't mean there aren't booms and busts, because that's the nature of technology. People get overexcited and then overly frustrated. So there are definitely some overbuilding issues, like the overbuilding of chips and power. I agree that the web is fundamentally different from computers in the way it's developed, and the adoption curve and all that stuff is going to be different.
Marc Andreessen:This also gets to my best theory of how the industry will play out, which is the question of how the industry will unfold, like whether there will be a few "god models" or a large number of models of varying sizes.
In the early days of the computer industry, like the original IBM mainframe, computers were very large and expensive, and there were only a few of them. For a long time, the general view was that this would be all there was to computers. Thomas Watson, the founder of IBM, famously said that he thought there would only be five computers in the world.
I think he meant that the government would have two, the three major insurance companies would have three, and then there would be nothing else that needed so much computing power. Computers were very large and expensive, so who could afford them? Who could afford the people needed to maintain them?
These computers were so large that they required special buildings to house them and people in white coats to maintain them because everything had to be kept very clean or the computer would stop working.
Today we have the concept of "god models" for AI, large base models, and back then we had the concept of god mainframes, with only a few of these computers. If you look at early science fiction, there was almost always this premise that there was a big supercomputer that either did the right thing or the wrong thing, and if it did the wrong thing, the plot of the sci-fi movie was usually that you had to go in and fix it or defeat it.
This top-down, single concept held true for a long time, especially before computers started getting smaller. Then later on came the so-called minicomputers, and the price went down from $50 million to $500,000, but even $500,000 was expensive, and the average person wouldn't have a minicomputer in their home, so a medium-sized company could buy a minicomputer, but not an individual.
Then with the advent of the PC, the price went down to $2,500, and with the advent of the smartphone, the price went down to $500. Today, you have computers of all shapes, sizes, and descriptions, and it might cost a penny, and it might be some embedded ARM chip and firmware, and there are billions of these computers.
Buy a new car today, and there might be 200 computers in it, or even more. Today you assume that everything has a chip, assume that everything needs a battery or electricity because they need to power the chip, and assume that everything is on the Internet because all computers are assumed to be on the Internet or will be on the Internet.
So the computer industry today is a huge pyramid, there are still a few supercomputer clusters or mainframes, which are the god models, and then there are more small computers, there are more PCs, there are more smartphones, and then there are a lot of embedded systems.
It turns out that the computer industry is a collection of all of these things. What kind of computer you need depends on what you want to do, who you are, and what you need.
If this analogy holds, it means that we will actually have AI models of all shapes, sizes, descriptions, capabilities, trained on different data, running at different scales, with different privacy policies, different security policies, there will be huge diversity and variation, and it will be an entire ecosystem, not just a few companies. What do you think of this view?
Ben Horowitz: I think it's right. I also think that another interesting thing about this era of computing is that if you look at previous eras of computing, from mainframes to smartphones, a huge source of lock-in is the difficulty of using them.
No one got fired for buying IBM because you had people who were trained and knew how to use the operating system and because of the huge complexity of dealing with computers, choosing IBM was a safe choice.
Even with smartphones, why are Apple smartphones so powerful because the cost and complexity of switching away from it is very high. AI is the easiest computer to use, it speaks English and talks to people.
Where is the lock-in? Do you have complete freedom to choose the size, price, speed that is right for your specific task, or are you locked into the God model? I think that's an open question, but very interesting, and it may be very different from previous eras of computing.