Written by: Biteye core contributor Viee Editor: Biteye core contributor Crush
Are you willing to hand over ten years of personal conversation data to OpenAI, Google or Facebook?
Imagine that the AI assistant of the future can perfectly replicate the way you think and handle daily affairs just like you. While exciting, this also means that AI needs to obtain a lot of data - including every message you have sent in the past, and all the information that makes up your unique personality, which raises the question raised at the beginning of the article. According to the survey, 59% of consumers are uneasy about using personalized AI, mainly because of concerns about data privacy.
Nillion, as an innovative decentralized network, provides a practical solution to this problem by utilizing multi-party computing (MPC) and other privacy-enhancing technologies (PET). Biteye will introduce you to the new concept of blind computing in Web3 and how it protects your and my data privacy.
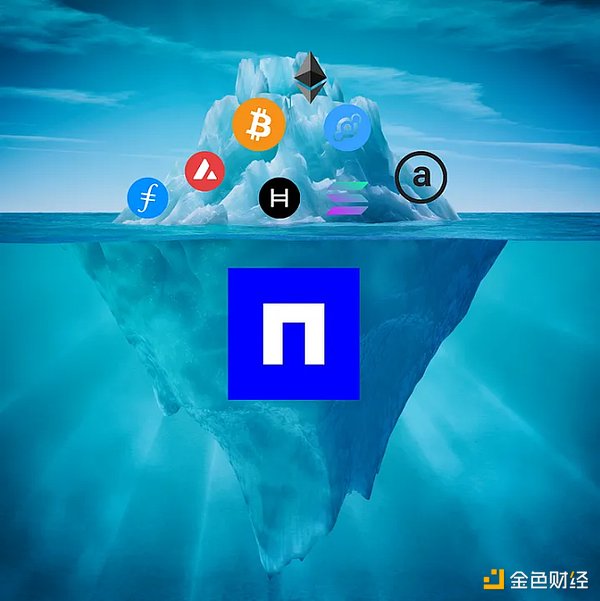
01 The current status of data privacy and security
Data is regarded as the new "oil" in the digital age, and privacy and security issues are becoming increasingly important. Traditional data processing methods usually require decrypting data before calculation, which exposes sensitive information to potential security threats during processing. For example, in the medical industry, patients' data must undergo strict privacy protection measures, but may still face the risk of leakage when analyzing. This not only affects users' trust in the service, but also limits the possibility of data sharing and collaborative research.
Although personalized AI shows great potential, data privacy issues must be taken seriously before realizing this vision, so that personalized AI can truly usher in the era of the "next Internet".
02 What is blind computing?
Nillion has proposed a new method to solve the above problems, that is "Blind Computing". It uses a decentralized network architecture and advanced privacy enhancement technology to enable high-value data to be securely stored and calculated without decryption.
Blind Computing allows users to perform calculations without direct access to the original data. This means that even if the data is stored in an untrusted environment, users can operate safely.
It mainly includes several processes:
The data is shielded and divided into several parts
These fragments are sent to different nodes
Nodes process data without seeing it
Collect and combine results
Only authorized parties can see the final output
In other words, the core of blind computing is to encrypt data before processing. Specifically, users encrypt data and send the encrypted data to cloud servers or other computing platforms. On these platforms, all calculations are performed on encrypted data, and the final result is also encrypted. After receiving the result, the user can get the final answer through the decryption process without knowing any information in the intermediate process. Like an "invisible computing assistant", "blind computing" got its name.
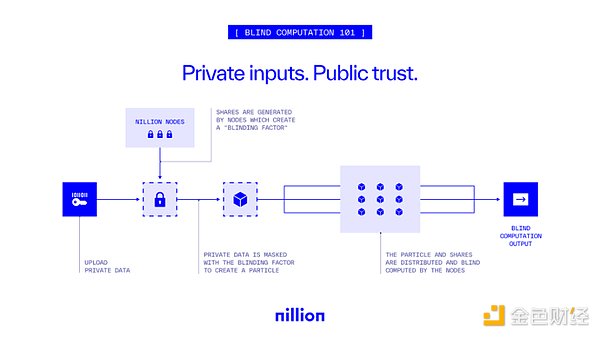
Blind computing combines a variety of advanced technologies to ensure that sensitive information is always safe during processing:
1. Multi-party computing (MPC)
Multi-party computing is a technology that allows multiple parties to jointly calculate a function without revealing their respective input data. Each participant only knows his own input and final result, and cannot access the input of other participants.
The working principle of MPC can be understood by the classic millionaire story. The problem was first proposed by Andrew Yao in 1982. Suppose two millionaires want to know who is richer, but don't want to reveal their wealth. They can use MPC to jointly calculate who is richer through a series of cryptographic operations without revealing their specific wealth. This process ensures the information security between the parties while enabling cooperation.
This is achieved through a series of cryptographic operations that allow each party to input their net worth into a shared calculation. The calculation is structured in a way that only the comparison result (i.e. which millionaire is richer) is output without revealing any details about their respective net worth. This problem shows the power of MPC - it can preserve privacy while enabling collaborative calculations.
Applications: In blind computations, MPC ensures that participating nodes cannot see the original data even if the computation is performed in a cloud server or other untrusted environment. This approach is ideal for processing sensitive information such as financial transactions or medical records.
2. Homomorphic Encryption
Homomorphic encryption is a special form of encryption that allows encrypted data to be directly calculated without decryption. Users can perform various operations (such as addition and multiplication) in an encrypted state, and the final result is still encrypted. Users can use their own keys to decrypt and get the correct answer.
Application:Homomorphic encryption plays an important role in blind computing, allowing servers to perform operations on encrypted data without knowing the data itself. This technology makes data more secure when processed in a cloud environment.
3. Privacy Enhancement Technology (PET)
Privacy enhancement technology is a series of methods designed to improve the level of personal privacy protection, including anonymization, pseudo-anonymization, and data desensitization.
Application:In blind computing, these techniques can be combined with MPC and homomorphic encryption to further ensure the security and privacy of data during processing. For example, by anonymizing the input data, any participant can be prevented from identifying the source of the data.
4. Quantum Blind Computing
Quantum blind computing is a method of implementing blind computing using the principles of quantum computing. It allows users to perform encrypted calculations on quantum computers and protect the privacy of input and output data.
Application:Quantum blind computing is still in the research stage. Once implemented, it is expected to be able to handle more complex problems and may expand users' computing capabilities in cloud environments.
03 Nillion's dual network architecture
In order to integrate the above technologies to achieve blind computing, Nillion adopts a dual network architecture, consisting of a coordination layer (NilChain) and an orchestration layer (Petnet). This design ensures efficient data storage and processing while maintaining the security and privacy of the system.
1. Coordination layer (NilChain)
The coordination layer is responsible for managing payment operations in the network, including storage and blind computing. It ensures that all transactions proceed smoothly and that resources are allocated efficiently.
2. Orchestration layer (Petnet)
The orchestration layer uses privacy-enhancing technologies such as MPC to protect static data and implement blind computing on these data. Petnet ensures that even when data is shared between multiple nodes, it maintains a high level of security and privacy. This level provides developers with a flexible platform that enables them to build a variety of applications to meet different needs. 04 Nillion Current Progress On October 30, Nillion just announced the completion of a $25 million financing round, led by Hack VC and supported by Arbitrum, Worldcoin and Sei. So far, Nillion's total financing has reached $50 million.
Since its launch, Nillion has achieved some impressive performance data:
Number of validators: 247,660
Total data protected: 711 GB
Total number of challenged secrets: 120,254,931
Validators help maintain the security and integrity of data, and the growth in this number means that the Nillion Network is becoming stronger and more secure.
Current partners of Nillion include blockchain networks NEAR, Aptos, Arbitrum, Mantle, IO.net, Ritual, etc. Involving multiple fields, such as Ritual and Nesa, which are used for private model AI model training and reasoning; Rainfall, Dwinity, and Nuklai, which are used to store, share, and profit from AI training data; MIZU, which is used to create synthetic data and protect personal data. Virtuals Protocol, Capx AI, and Crush AI create personalized private agents with the help of Nillion. PINDORA is used for confidentiality and security support for the DePIN network. Nillion attempts to attract projects at the intersection of blockchain and AI that need to securely share and store large amounts of data.
In the future, we can foresee that Nillion will be widely used in multiple fields such as healthcare, finance, and education, contributing to building a more secure and transparent data ecosystem.
05 Summary
Nillion provides a feasible way to solve the data privacy problem in the current digital world through innovative technical architecture and strong privacy protection capabilities, allowing users to enjoy the convenience brought by digital services without worrying about the leakage or abuse of personal information.
At present, we cannot imagine the future of artificial intelligence. The rise of personalized digital copies and concerns about data privacy are like the two ends of a seesaw. Without effective data privacy protection measures, personalized AI will find it difficult to gain widespread market acceptance. Therefore, how to find a balance between promoting technological progress and protecting user privacy will be an important issue that the industry needs to solve urgently. With the development of the Nillion network, we look forward to seeing more new applications based on this platform, which will have a positive impact on human society in the AI era.