Author: Teng Yan, former head of NFT research at Delphi Digital; Translation: 0xjs@黄金财经
I am fascinated by the idea of decentralized collective intelligence, so recently, I have been exploring the Bittensor model to bootstrap AI networks in specific fields.
But I found that Bittensor has several serious flaws. My findings were shocking and gave me pause.
Bittensor Quick Review
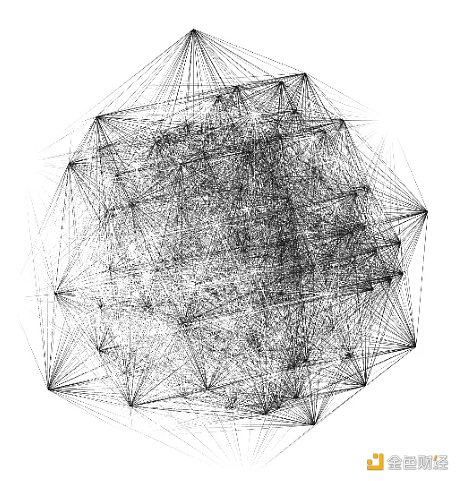
Source: Bittensor.com
Bittensor operates as a decentralized incentive network for AI models.
Across its 32 subnets, miners deploy AI models that compete based on specific criteria. These outputs are then evaluated and ranked by validators, and miners earn TAO tokens based on their relative performance. Currently, these subnets are working on tasks such as text generation, image generation, text-to-speech, and model fine-tuning.
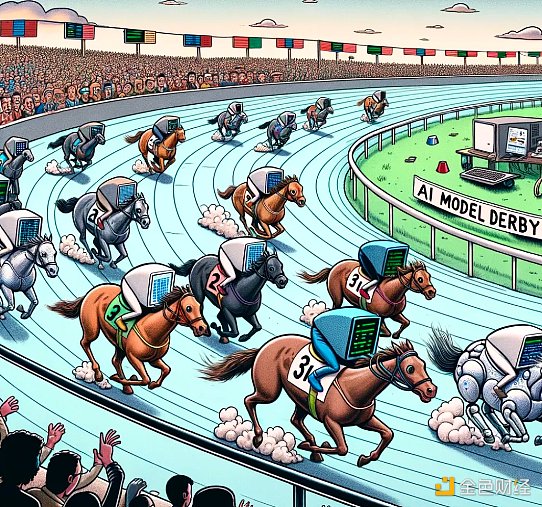
“Horse Race for AI Models”: Image generated using DALL-E 2
In short: it’s a horse race for AI models. The best horses (models) get the biggest rewards.
The important idea here is that monetary incentives naturally attract the best performing models and innovators to the most relevant subnets.
I first shared some of my early thoughts on Bittensor in November of last year. Since then, Bittensor has gradually become the darling of Crypto Twitter and the standard bearer of the decentralized AI movement. As of now, Bittensor has a market cap of $2.8 billion and a fully diluted valuation of $8.5 billion.
A Bumpy Road Ahead
In my opinion, the biggest problems with Bittensor are:
1. Broken Horizontal Economy
If implemented properly, incentive-driven competition within subnets to produce the best output makes sense.
Note the bold, capitalized "if".
There are challenges such as incentive gaming, relay mining, and validators' knowledge of the truly correct output. I believe these issues can be solved with enough research and experimentation. Some subnets have already taken measures or modified their standards to prevent relay mining and other improper behavior.
However, when competition expands horizontally, the economic model begins to break down.
Subnets must compete with each other for a larger share of inflation rewards. This will become more apparent once a dynamic TAO is implemented (subnets will have their own dynamic tokens, and token holders can influence the distribution of rewards between subnets)
This begs the question:
How should TAO holders compare the economic value that different subnets bring to the Bittensor Network? For example:
(1) a text-to-speech model (Subnet 3) vs.
(2) a vision model that screens for diseases in X-rays?
The value generated in each case is completely different. You can interpret this question in many ways, but the answer is never clear-cut. Relying on market forces to determine the value contribution of each subnet is not optimal for long-term strategic development.
It would be like having each AAPL shareholder vote on the next product Apple should develop.
2. Competitive challenges of subnets
I doubt that Bittensor subnets are competitive in basic generative AI tasks such as general image and text generation. There are already open source projects that excel in these areas, which are often driven by reputation, research contributions, and public service.
Take the powerful large language model (LLM) Llama-3 released as an open source tool by Meta. Although billions of dollars have been invested in its development, Llama-3 is still free to use and has received strong support from the community. Since its release last week, it has been downloaded more than 500,000 times on Huggingface.
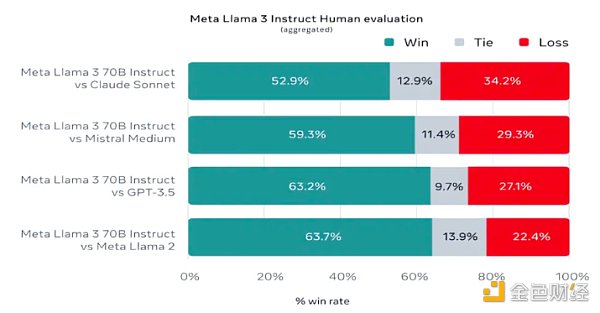
Benchmark comparisons show that open source LLMs like Llama-3 are already the best in their class, and are arguably on par with proprietary models like OpenAI's GPT-4.
To date, I have not seen Bittensor subnets achieve results or features that surpass existing open source models. Nor have I seen evidence that they outperform established benchmarks. At some point, you have to ask yourself if they will do so.
3. High cost of running the smart network
The network relies heavily on its native token TAO to run. Miners mainly join the network when they find computational arbitrage opportunities, especially when mining rewards exceed computational costs.
Demand for TAO depends on:
(1) Speculators (a whole can of worms we won’t get into here)
(2) Adding more Bittensor subnets, or increasing demand to become a miner or validator. However, this growth tends to disincentivize existing participants. Falling token prices will reflexively reduce network activity as miner/validator unit economics come under pressure.
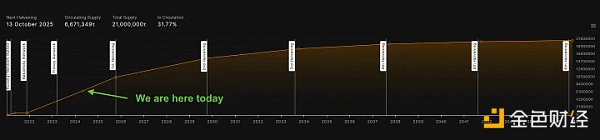
Source: Taostats.io
Bittensor is expensive to operate, paying 7,200 TAO inflation per day to subnet owners + miners + validators. This is equivalent to about $3.2 million per day or $1.2 billion per year.
This raises a key question: where does this money come from? Mainly speculators. But will Bittensor generate $1.2 billion in value in a year? Its valuation may have far exceeded its own value.
4. Transparency
While Bittensor offers a degree of decentralization, it is worth noting that it is not open source - a crucial distinction. While participation in the subnet is open to anyone, the internal mechanisms are not transparent, resulting in limited user visibility into the following activities:
What are the miners doing?
What algorithms do they run?
How are the final results produced?
This lack of transparency may limit the diversity of applications developed using subnet outputs, as many developers need to clearly understand the underlying reasoning process.
Some Thoughts
I am not against Bittensor. In fact, I find it to be one of the most interesting experiments in the emerging Crypto x AI field.
It has successfully gathered a group of smart people around its vision. It may inspire many future projects. However, Bittensor must face the current challenges head-on to have a chance to succeed in the real world, otherwise it will be lost in the grave of speculative hype.
Some closing thoughts:
Greater synergies could be achieved if all subnetworks within the network were targeted to specific sectors rather than being scattered across various unrelated tasks.
For example, I envision "Bittensor for genomic research/drug discovery" or "Bittensor for finance/trading". This could actually work quite well. Such alignment of vision could foster economic cohesion, strategic direction, and business development efforts.
Bittensor's economic model (in its current state) is probably best suited for AI niches that don't attract widespread attention but could benefit from targeted incentives. Potential applications include cryptocurrency-specific tasks such as predicting token prices.
Finally, I strongly believe that decentralization of AI has an extremely important role to play in the world - to balance the global dominance of large tech companies and to foster innovation, especially through open source initiatives. I will elaborate on my thoughts in a future post.