Author: Zhao Jian
On January 27, two years ago, "Jiazi Guangnian" participated in a salon on the theme of AI-generated videos. There was an interesting interaction at the meeting: How soon will AI video generation usher in the "Midjourney moment"?
The options are within half a year, within one year, 1-2 years or longer.
Yesterday, OpenAI announced the exact answer: 20 days.
OpenAI yesterday released Sora, a new AI-generated video model. With performance advantages visible to the naked eye and a video generation time of up to 60s, following the text (GPT-4 ) and images (DALL·E 3), it has also achieved "far leadership" in the field of video generation. We are one step closer to AGI (Artificial General Intelligence).
It is worth mentioning that the star AI company Stability AI originally released a new video model SVD1.1 yesterday, but due to a collision with Sora, its official push The article has been quickly deleted.
Cristóbal Valenzuela, co-founder and CEO of Runway, one of the leaders in AI video generation, tweeted: "The game is on."< /p>
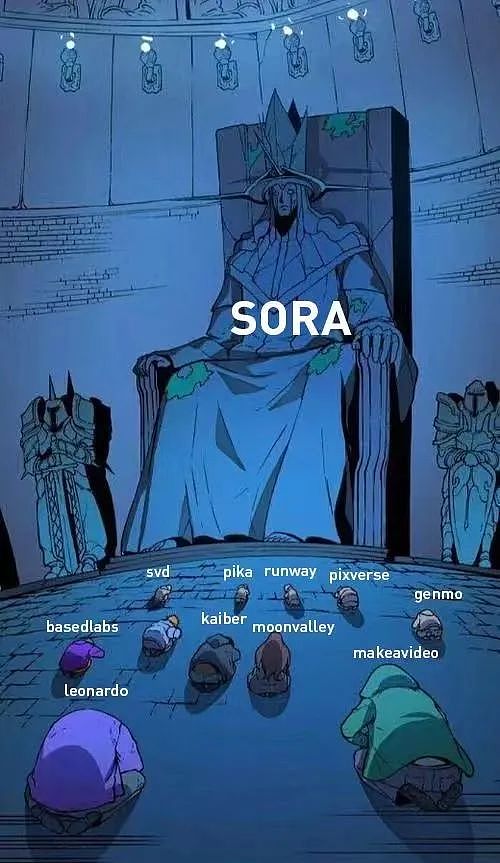
OpenAI also released a technical document yesterday, but in terms of model architecture and training methods, it did not release any genius-level innovative technology, but more optimization of existing technology routes.
But like ChatGPT, which was launched more than a year ago, the secret of OpenAI is the tried and tested Scaling Law - when the video model is enough " "Big" will produce the ability for intelligence to emerge.
The problem is that almost everyone knows the "violent aesthetics" of large model training. Why is it OpenAI again this time?
1. The secret of data: from token to patch
The technical route for generating videos has mainly gone through four stages: recurrent networks (RNN), generative adversarial networks (GAN), autoregressive transformers, and diffusion models.
Today, most of the leading video models are diffusion models, such as Runway, Pika, etc. Autoregressive models have also become a popular research direction due to their better multi-modal capabilities and scalability, such as VideoPoet released by Google in December 2023.
Sora is a new diffusion transformer model. As can be seen from the name, it combines the dual characteristics of the diffusion model and the autoregressive model. The Diffusion transformer architecture was proposed in 2023 by William Peebles of the University of California, Berkeley, and Saining Xie of New York University.
How to train this new model? In the technical document, OpenAI proposed a way to use patches (visual patches) as video data to train video models, which is inspired by the tokens of large language models. Tokens elegantly unify multiple modes of text—code, mathematics, and various natural languages—while patches unify images and videos.
OpenAI trains a network to reduce the dimensionality of visual data. This network receives raw video as input and outputs a latent representation that is compressed in both time and space. Sora is trained on this compressed latent space and subsequently generates videos. OpenAI also trains a corresponding decoder model that maps the generated latent representation back to pixel space.
OpenAI stated that past image and video generation methods often resize, crop or trim the video to standard dimensions, which loses the quality of the video generation. For example, a 4-second video with a resolution of 256x256. After the image and video data are patched, the original data of videos and images with different resolutions, durations and aspect ratios can be trained without compressing the data.
This data processing method brings two advantages to model training:
< strong>First, sampling flexibility. Sora can sample widescreen 1920x1080p video, vertical 1080x1920 video and everything in between, creating content directly for different devices in their native aspect ratio, and quickly to prototype content at a lower size. These all use the same model.
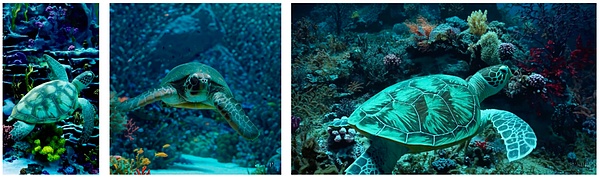
Second, improve the frame and composition. OpenAI has empirically found that training on videos in their original aspect ratio improves composition and framing. For example, a common model that crops all training videos into squares sometimes produces videos with only partially visible subjects. In contrast, Sora's video framing is improved.
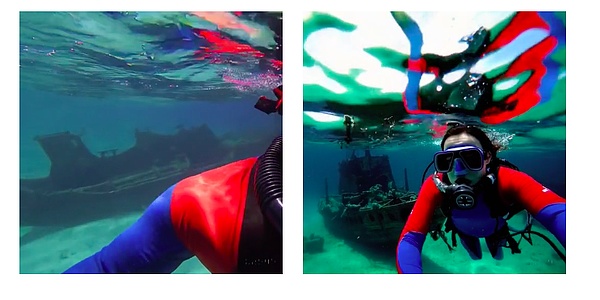
Model trained on square crops (left), Sora's model (right)
At the language understanding level, OpenAI found that training on highly descriptive video captions improves text fidelity and the overall quality of the video.
To this end, OpenAI applied the "re-captioning technique" introduced in DALL·E 3 - first training a highly descriptive A subtitle generator model and then use it to generate text subtitles for the videos in the training dataset.
In addition, similar to DALL·E 3, OpenAI also uses GPT to convert short user prompts into longer detailed subtitles and then send them to the video model. This enables Sora to generate high-quality videos that accurately follow user prompts.
Prompt words: a woman wearing blue jeans and a white t-shirt, taking a pleasant stroll in Mumbai India during a colorful festival.
In addition to text-generated video, Sora also supports "image-generated video" and "video-generated video".
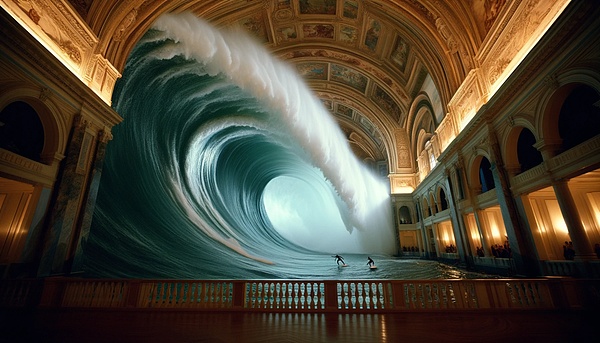
Prompt words: In an ornate, historical hall, a massive tidal wave peaks and begins to crash. Two surfers, seizing the moment, skillfully navigate the face of the wave.
This feature enables Sora to perform a variety of image and video editing tasks, creating perfect looping videos, animated still images, extending videos forward or backward in time, and more.
2. The secret of computing: still the "aesthetics of violence"
In Sora's technical documentation, OpenAI did not disclose the technical details of the model (Elon Musk once criticized OpenAI as no longer what it was originally intended to be when it was founded) open"), but only expresses a core concept-scale.
OpenAI first proposed the secret of model training in 2020-Scaling Law. According to Scaling Law, model performance will continue to improve like Moore's Law based on large computing power, large parameters, and big data. It is not only applicable to language models, but also to multi-modal models.
OpenAI followed this set of "violent aesthetics" to discover the emergent ability of large language models, and eventually developed the epoch-making ChatGPT.
The same goes for the Sora model, which, with Scaling Law, hit video’s “Midjourney moment” in February 2024 without warning.
OpenAI stated that transformer has demonstrated excellent scaling properties in various fields, including language modeling, computer vision, image generation, and video generation. The figure below shows that during the training process, with the same samples, as the training calculation scale increases, the video quality improves significantly.
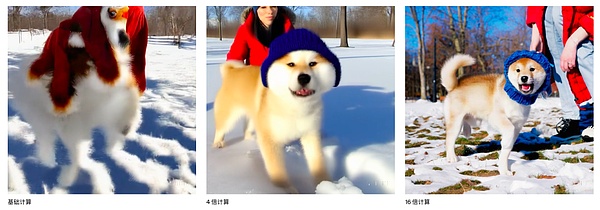
OpenAI found that video models exhibit many interesting emerging capabilities when trained at scale, allowing Sora to simulate certain aspects of real-world people, animals, and environments. These properties appear without any explicit inductive bias to 3D,objects etc. - purely a model scaling phenomenon.
Therefore, OpenAI names the video generation model "world simulators" (world simulators), or "world model" - which can be understood as Let machines learn the same way humans understand the world.
NVIDIA scientist Jim Fan commented: "If you think OpenAI Sora is a creative toy like DALL·E...think again . Sora is a data-driven physics engine. It is a simulation of many worlds, both real and fantasy. The simulator learns complex rendering, 'intuitive' physics, long-term reasoning and semantics with some denoising and gradient math Basics."
Meta Chief Scientist Yann LeCun proposed the concept of world model in June 2023. In December 2023, Runway officially announced its universal world model, claiming that it would use generative AI to simulate the entire world.
OpenAI only gives Sora the ability to model the world through the Scaling Law that it has long been familiar with. OpenAI says: "Our results show that extending video generative models is a promising route to building general simulators of the physical world."
Specifically, the Sora world model has three characteristics:
3D consistency. Sora can generate videos with dynamic camera movements. As the camera moves and rotates, people and scene elements move in unison in three-dimensional space.
RemoteRelevanceand object persistence. A significant challenge for video generation systems is maintaining temporal consistency when sampling long videos. OpenAI found that Sora is often (though not always) effective at modeling short- and long-term dependencies. For example, models can retain people, animals, and objects even if they are occluded or leave the frame. Likewise, it can generate multiple shots of the same character in a single sample and maintain its appearance throughout the video.
Interact with the world. Sora can sometimes simulate actions that affect world conditions in simple ways. For example, a painter can leave new brushstrokes on a canvas that persist over time.
Analog the digital world. Sora is also capable of simulating manual processes - one example is video games. Sora can simultaneously control players in Minecraft through basic strategies while rendering the world and its dynamics with high fidelity. These abilities can be zero-shot by prompting Sora with a title that mentions Minecraft.
However, like all large models, Sora is not a perfect model yet. OpenAI acknowledges that Sora has many limitations and that it cannot accurately simulate many fundamental interacting physical processes, such as glass breaking. Other interactions (such as eating food) do not always produce the correct change in the object's state.
3. Is computing power the core competitiveness?
Why can OpenAI rely on "Scaling Law" to succeed repeatedly, but other companies have not?
We may be able to find many reasons, such as belief in AGI, persistence in technology, etc. But a practical factor is that Scaling Law requires high computing power expenditure to support, which is what OpenAI is good at.
In this way, the competition point of video models is somewhat similar to language models. The first is the team’s engineering parameter adjustment capabilities, and the last is the computing power. .
In the final analysis, this is obviously another opportunity for Nvidia. Driven by this round of AI craze, NVIDIA's market value has been rising steadily, surpassing Amazon and Google in one fell swoop.
The training of video models will consume more computing power than language models. With the global shortage of computing power, how does OpenAI solve the computing power problem? If combined with previous core-making rumors about OpenAI, everything seems to fall into place.
Since last year, OpenAI CEO Sam Altman (Sam Altman) has been raising 8 billion to 100 million for the chip manufacturing project code-named "Tigris". With US$100 million in funding, it hopes to produce an AI chip similar to Google's TPU that can compete with Nvidia to help OpenAI reduce operating and service costs.
In January 2024, Altman also visited South Korea and met with executives from South Korea's Samsung Electronics and SK Hynix to seek cooperation in the chip field.
Recently, according to foreign media reports, Altman is promoting a project aimed at improving global chip manufacturing capabilities and is working with the United Arab Emirates government, including Negotiations with different investors. The funds raised by this plan have reached an exaggerated US$5 trillion to US$7 trillion.
An OpenAI spokesperson said: "OpenAI has had productive discussions about increasing the global infrastructure and supply chain for chips, energy and data centers that are critical to artificial intelligence. and related industries are critical. Given the importance of national priorities, we will continue to brief the U.S. government and look forward to sharing more details at a later date."
NVIDIA founder and CEO Jensen Huang responded slightly sarcastically: "If you think computers can't develop faster, you might come to the conclusion that we need 14 planets, 3 galaxies, and 4 suns to do it. All this provides fuel. However, computer architecture is actually constantly improving."
Is it the development of large models that is faster, or the reduction of computing power costs? Faster? Will it be the winner in the 100-model war?
In 2024, the answer will gradually be revealed.
p>