Author: Alphatu Source: X, @Alphatu4 Translation: Shan Oppa, Golden Finance
Since OpenAI began rolling out new voice and image features on its ChatGPT platform in September 2023, a more intuitive interface has been introduced to allow users to voice conversations and share with ChatGPT images, thereby enhancing the overall user experience.
This situation has further exacerbated the already booming popularity of intermodal transportation.
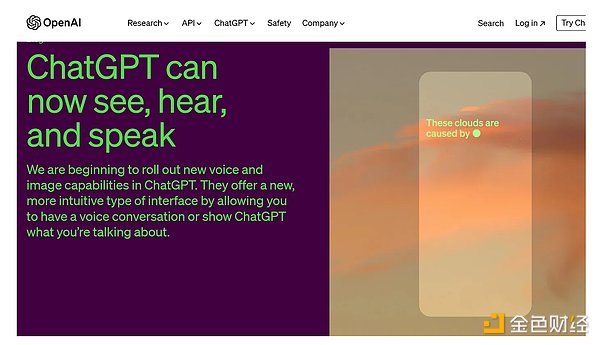
p>
In fact, the integration of voice and image functions provides users with multiple ways to interact with ChatGPT in all aspects of life. Whether on the go or at home, users can now leverage these multimodal capabilities for more immersive interactions with AI models, adding imagination to many product scenarios that were previously impossible.
Multimodality will be more widely used in industrial scenarios than general language models.
What is multimodal artificial intelligence?
Multimodal artificial intelligence refers to artificial intelligence systems and models that can understand and process information from multiple modes or sources. In the context of artificial intelligence, a modality is a different form or channel of input, such as text, images, audio, video, or any other type of data. Multimodal AI aims to integrate and analyze information from various modalities to achieve a more comprehensive understanding of the data.
The widespread use of graphics processing units (GPUs or TPUs) has greatly promoted the development of deep learning AI. Generative AI, however, takes this progress even further, giving it the seemingly insatiable ability to absorb data in the form of tokens, and parameters that represent the number of connections between neurons. Additionally, it utilizes a computing power metric called Floating Point Operations (FLOPS). The latest GPT-4 model is now equipped with multi-modal capabilities, can blend text and images, and has been significantly enhanced, winning praise for its superior performance over existing LLMs on a variety of natural language processing tasks.
Multimodal artificial intelligence and industrial scenarios
However, the constraints of single-modal data This brings challenges to real-life scenarios, especially industrial scenarios, and requires the use of multi-modal artificial intelligence.
In information-rich scenarios, relying solely on "language" models is not enough. Effective decision-making and information evaluation require multiple signals.
Take the manufacturing industry as an example. There is a large amount of image, temperature, weight and other data in the manufacturing industry. In this case, relying solely on language models is not enough, which highlights the need to integrate various forms of information.
Take the medical field as an example. Why do doctors prefer face-to-face diagnosis, and why can’t current artificial intelligence fully diagnose diseases? The explanation lies in the doctor analyzing the text and the patient's performance. When examining a specific X-ray, doctors engage in brainstorming and consultation as they extract more than just an image or passage of text, interpreting multimodal information.
Multimodal input is not limited to text, but also includes sound, infrared data and other elements. This approach helps train models to think in multiple dimensions.
Consider a self-driving car equipped only with a camera system; it will have difficulty identifying pedestrians in low-light conditions. To fully address these challenges, the combination of lidar, radar and GPS is critical. This integration enables vehicles to more fully perceive their surroundings, thereby improving driving safety and reliability.
The underlying principles here emphasize the importance of integrating multiple senses to gain a deeper understanding of complex events. By leveraging multimodal AI, textual information, photos, videos, and audio can be fused to form a coherent and comprehensive description of a given situation.
Artificial intelligence fundamentally solves knowledge problems, while the Internet mainly solves information problems. Knowledge is domain-specific in nature and lacks the universality of the Internet. The collaborative integration of domain experts and multimodal AI capabilities within manufacturing has the potential to significantly reduce costs and increase efficiency.